Your constraint that $\|f\|^2_2 = \sum_{j,k} |\langle f, \psi_{j,k} \rangle|^2, $ is too strong. That constraint does guarantee a tight frame, but moreover a Parseval one. (And Melody's proof shows that a frame which is simultaneously Parseval and normalized must be an ONB.)
A tight frame is one where the optimal upper and lower frame bounds equal each other. That is, there exist $A$ and $B$ such that
$$A\|f\|^2_2 \le \sum_{j,k} |\langle f, \psi_{j,k} \rangle|^2 \le B\|f\|^2_2$$
with $A=B$.
A Parseval frame is one where $A=B=1$.
Note: Any $A$ and $B$ which satisfy the expression above are called frame bounds, regardless of whether they are optimal or not. The optimal frame bounds will be the smallest and largest singular values from the SVD when applied to the matrix containing the vectors.
Further, any tight frame with the same number of frame vectors as the dimension of the space will become Parseval when the vectors are normalized (and thus will become an ONB). To get a tight, normalized frame which is not an ONB, we need any tight frame with more vectors than dimensions of the space, and normalize them.
For $L^2(\mathbb R)$, the so-called Mercedes-Benz frame will do:
$$\Phi = \left[ \begin{array}{rrr}
0 & \frac{1}{\sqrt 2} & -\frac{1}{\sqrt 2} \\
\sqrt\frac{2}{3} & -\frac{1}{\sqrt 6} & -\frac{1}{\sqrt 6} \\
\end{array} \right]$$
This frame has (both upper and lower) frame bounds 1, and is thus Parseval.
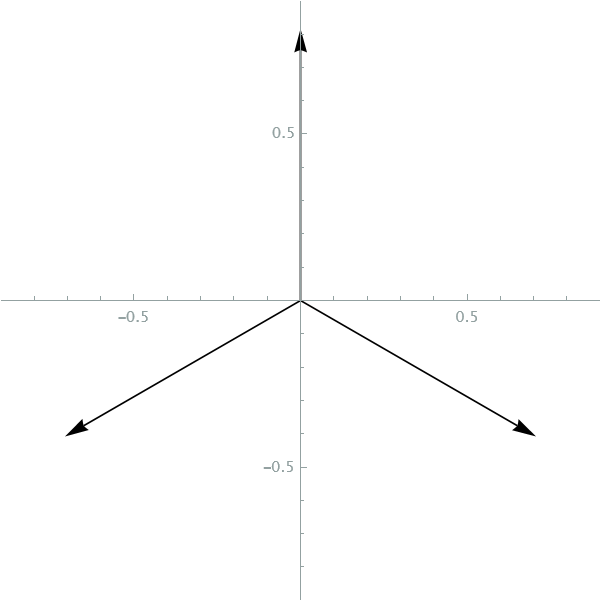
We multiply it by $\sqrt\frac{3}{2}$ to normalize it:
$$\Psi = \left[ \begin{array}{rrr}
0 & \frac{\sqrt 3}{2} & -\frac{\sqrt 3}{2} \\
1 & -\frac{1}{2} & -\frac{1}{2} \\
\end{array} \right]$$
The normalized $\Psi$ now has (both upper and lower) frame bounds $\sqrt\frac{3}{2}$ and thus is still tight (but no longer Parseval). Both $\Phi$ and $\Psi$ are tight frames and neither is an ONB, but $\Psi$ also satisfies your normalized criteria.