(Too long for a comment.)
Numerical experiments suggests that one cannot do better than $1 / \sqrt{\pi x}$ (or one of the equivalent variants, the set of minimizers appears to be quite large). Here is a plot of three minimizers obtained numerically for the discrete analogue of the problem on $\{0, 1, 2, \ldots, n - 1\}$ with $n = 75$. These minimizers were found by Mathematica with three different numerical optimization methods (blue: "DifferentialEvolution"
, yellow: "NelderMead"
, green: "SimmulatedAnnealing"
). The corresponding norms are 1.12145, 1.12842, 1.1265, respectively.
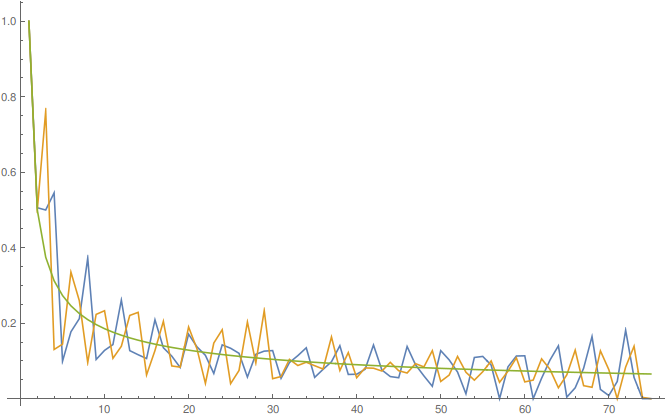
Mathematica code:
n = 75;
expr = Sum[x[i], {i, 0, n - 1}]/Sqrt[n];
constr = Join[
Table[Sum[x[j] x[i - j], {j, 0, i}] >= 1, {i, 0, n - 1}],
Table[x[i] >= 0, {i, 0, n - 1}]];
vars = Table[x[i], {i, 0, n - 1}];
{min1, subst1} =
NMinimize[{expr, constr}, vars, Method -> "DifferentialEvolution"];
{min2, subst2} =
NMinimize[{expr, constr}, vars, Method -> "NelderMead"];
{min3, subst3} =
NMinimize[{expr, constr}, vars, Method -> "SimulatedAnnealing"];
{min1, min2, min3}
ListPlot[{vars /. subst1, vars /. subst2, vars /. subst3},
Joined -> True, PlotRange -> All]